Understanding Models: Their Definition and Impact
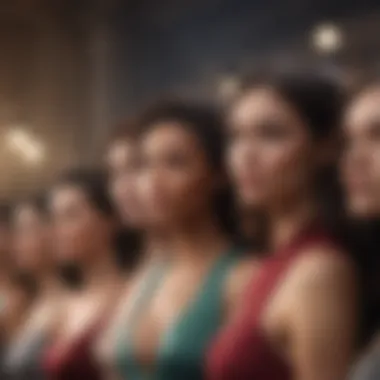
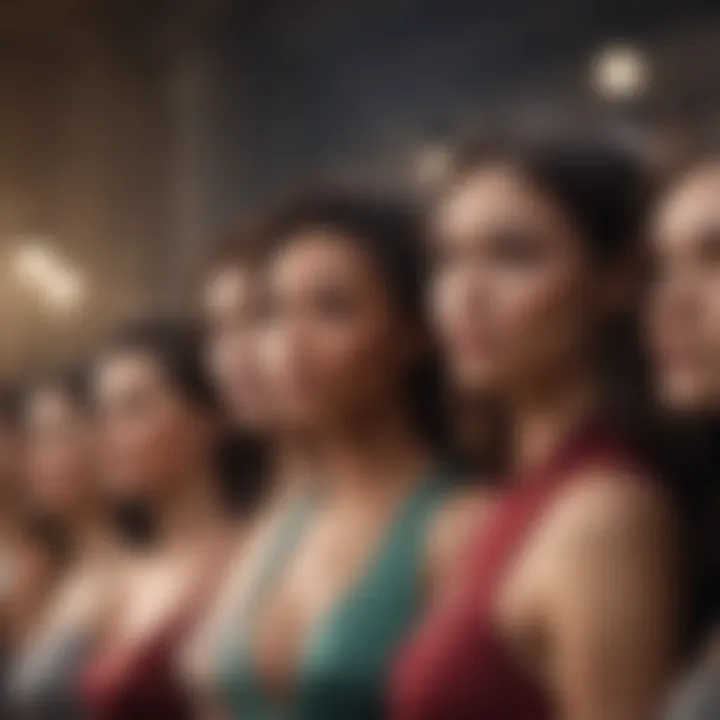
Intro
The term "model" resonates across various disciplines, often embodying a fundamental concept that aids in understanding complex systems. In this discussion, we aim to dissect the multifaceted nature of models, shedding light on their definitions, significance, and practical implications. From the sterile laboratories of science to the bustling world of business, models serve as essential tools that guide decision-making, strategy formulation, and predictive analytics.
Importance of Models
At their core, models should not merely be viewed as representations or imitations. They act as simplified abstractions of reality that help illuminate the intricate web of variables interplaying in our surroundings. Whether it's a mathematical formula predicting economic outcomes or a diagram illustrating the flow of information in a corporate ecosystem, models find their place wherever complexity needs distillation. They enable us to make informed choices, paving pathways to insights that would be otherwise obscured by chaos.
"Models serve as critical instruments in deciphering the complexities of our world, transforming data into actionable insights."
In numerous domains, models evolve continuously, adapting to innovations, shifts in paradigms, and emergent theories. They often transcend their initial constructs, leading to new findings or perspectives, enhancing their role as invaluable tools in research and application alike.
With this foundation laid, we now turn our attention to the first header.
Defining a Model
To lay the groundwork for the examination of models, we must first grasp what constitutes a model in both simple terms and elaborate contexts. A model is essentially a simplified representation of reality—a roadmap or blueprint that aids in understanding complex phenomena. By breaking down intricate systems into more digestible parts, models enable us to analyze, predict, and communicate ideas effectively.
Understanding models is pivotal not just in academics but extends to everyday life. For a financial enthusiast or an individual investor, models can serve as critical tools for decision-making, risk assessment, and strategic planning. They provide clarity amidst chaos, allowing one to visualize potential outcomes and navigate uncertainties with more confidence.
Fundamental Characteristics
Models possess fundamental characteristics that distinguish them from mere approximations. These consist of:
- Simplicity: At their core, models aim to simplify complexity. Whether it's a financial forecast or a biological process, a model channels the essential features while eliminating superfluous details.
- Functionality: Each model serves a specific objective. For example, an investor might use a financial model to evaluate multiple investment strategies, focusing on returns and risks.
- Representational Nature: Models symbolize certain aspects of reality. They can be visual (like graphs), mathematical (like equations), or conceptual (like frameworks). This representation helps bridge theoretical concepts and practical applications.
- Scalability: Many models can operate on various levels, whether applied to micro (individual) or macro (aggregate) contexts, thus retaining relevance across different scenarios.
In any sophisticated discussion, acknowledging these traits allows for a nuanced interpretation of models. They aren’t just static entities; they evolve and grow, just as our understanding of the world does.
Different Contexts of Use
Models find application in myriad fields, revealing their versatility and importance. Some notable contexts include:
- Economics: Economic models employ various assumptions to predict how markets or economies behave. The classic example is the supply and demand model, which illustrates how price changes can affect consumer behavior.
- Healthcare: In health services, models predict the spread of diseases, helping in planning responses to pandemics. This could involve statistical models analyzing infection rates and healthcare resource allocation.
- Business: Companies utilize models to tackle strategic planning. Business models define the value proposition and operational approach of a company, shaping decisions from product development to marketing strategies.
- Environmental Science: Scientists depend on ecological models to understand environmental dynamics and explore the impacts of human activities on ecosystems. These models help in sustainability efforts and conservation planning.
These examples underscore the multifaceted focus of models in varying sectors. Recognizing that models are not one-size-fits-all serves as a foundation for deeper exploration into types and applications across various disciplines.
Types of Models
In the realm of analysis and interpretation, the classification of models into distinct categories provides a structured way to comprehend their varying purposes and characteristics. Understanding the different types of models is vital, as it aids in selecting the appropriate model for a specific situation, determining expected outcomes, or even guiding research. This section will delve into several types of models that hold significance across various disciplines, illuminating their unique characteristics and practical implications.
Physical Models
Physical models are tangible representations designed to replicate objects or systems. They range from architectural scale models to globes of Earth or anatomical models used in medical education. Notably, physical models offer several advantages. They enable users to visualize complex structures and processes, thereby simplifying comprehension. For instance, a three-dimensional model of a human heart can facilitate better understanding of its anatomy and functioning compared to a flat diagram. However, limitations such as scale and material differences can sometimes impede accuracy.
A physical model often acts as a stepping stone to deeper insights, grounding abstract concepts into something more graspable.
Mathematical Models
Mathematical models use mathematical language to describe a system or process. They can take various forms, from equations and graphs to algorithms. Mathematical models are particularly advantageous due to their ability to predict outcomes and analyze patterns by utilizing quantitative data. For example, investors often make use of financial models to forecast the future performance of stocks or market trends. However, the reliance on assumptions and simplifications can sometimes lead to inaccuracies or misinterpretations.
Conceptual Models
Conceptual models represent abstract ideas or systems through diagrams, frameworks, or theoretical constructs. These models serve to clarify notions or guide reasoning within a particular field. For instance, Bloom's Taxonomy in education categorizes cognitive skills, helping educators design curricula. The strength of conceptual models lies in their ability to distill complex ideas into a coherent framework, but they may sometimes oversimplify reality, neglecting nuances and interactions.
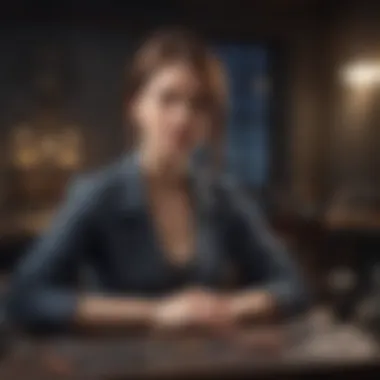
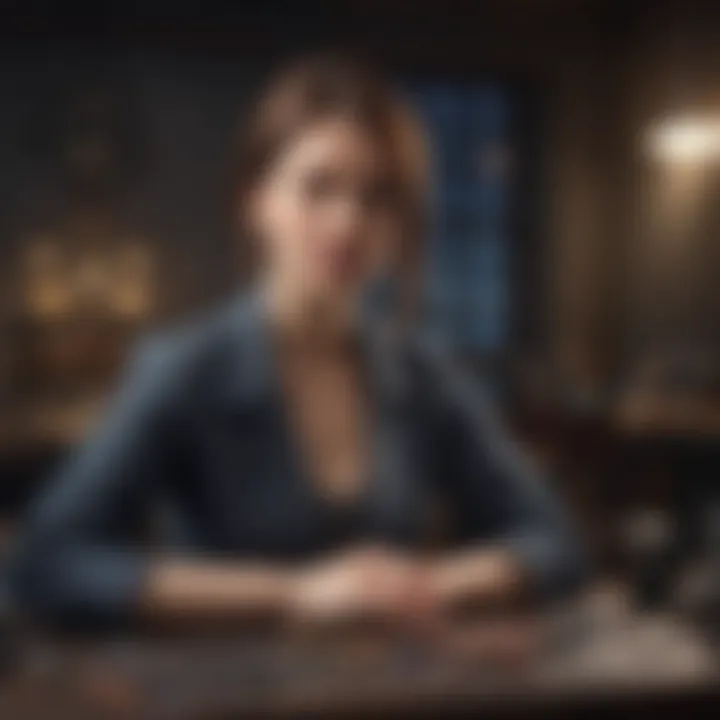
Simulation Models
Simulation models employ computer programs to create a digital representation of a real-world system. These models allow for experimenting with various scenarios to observe potential outcomes. In fields like meteorology, simulation models can predict weather patterns based on numerous variables. They provide a dynamic way of understanding processes over time, although the accuracy of simulation outcomes can heavily depend on the quality of input data and assumptions made during the modeling phase.
Statistical Models
Statistical models utilize statistical methods to analyze and interpret data for making inferences or predictions about a population based on a sample. Commonly seen in research and business analytics, these models help reveal correlations and relationships between variables. For example, a regression model can show the relationship between consumer spending and various economic factors. While they are powerful tools for data interpretation, statistical models can lead to misleading conclusions if the underlying assumptions are not met or if the data is flawed.
Understanding these types of models equips individuals with the knowledge needed to approach problems thoughtfully, select the right tools, and develop insights based on solid reasoning. As we venture further into the realms of theoretical frameworks, applications, and development processes in the following sections, the versatility and significance of each model type will become even clearer.
Theoretical Frameworks and Models
The concept of theoretical frameworks and models plays a pivotal role in enhancing our understanding of various phenomena across multiple disciplines. By providing structured approaches to hypothesize, analyze, and interpret intricate ideas, these frameworks serve as essential tools in both theoretical pursuits and practical applications. They streamline complexity and ground research in a set of principles that facilitate clear communication of concepts, ideas, and methodologies. Moreover, frameworks assist researchers and academics in articulating the relationships and dynamics observed within their studies. In essence, they are not just decorative labels; they provide the scaffolding necessary for intellectual exploration.
Role in Scientific Inquiry
The essence of scientific inquiry leans heavily on frameworks and models, which serve as the backbone of research efforts. When embarking on a study, scientists often start by establishing a theoretical foundation. This foundation allows them to pose questions that can lead to significant discoveries. For instance, in the realm of physics, Newton’s laws act as a foundational model for understanding motion. These laws provide a guide for scientists to predict outcomes under certain conditions, firmly rooting their inquiries in established parameters.
Moreover, theoretical models can also catalyze new hypotheses. They lay down a clear path for researchers to explore variables and their interactions. For example, in the field of psychology, the behaviorist model prioritizes observable behaviors, leading to hypotheses focused on stimulus-response patterns. Without such frameworks, the scientific community would lack coherence, making it difficult to build upon previous knowledge.
"A framework gives shape to inquiry, transforming vague ideas into testable propositions."
Impact on Hypothesis Development
Building upon a theoretical framework is critical for hypothesis development, acting as a bridge between raw observations and refined scientific testing. Research often begins with observations that spark curiosity. Converting those observations into testable hypotheses requires an underlying model that links the observed phenomena to theoretical concepts. For instance, in studying economic trends, one might draw from Keynesian theory to form a hypothesis regarding the impact of government spending on employment rates.
Furthermore, the integration of various models can enrich hypothesis formulation. When researchers consider interdisciplinary approaches, combining insights from different fields can illuminate new avenues for investigation. This can be seen in public health research, where models from epidemiology and behavioral science converge to formulate hypotheses about disease spread and prevention strategies.
Applications in Various Fields
The utilization of models manifests in myriad ways across an array of disciplines, shaping decision-making processes, enhancing problem-solving effectiveness, and enriching theoretical understanding. In this section, we will examine how models serve as essential tools in different fields, providing clarity in complexity and fostering connections between abstract concepts and tangible outcomes. By analyzing the specific benefits and considerations of various applications, we highlight their pivotal role in contemporary practice.
Business and Management Models
In the realm of business, models are foundational for strategic planning and operational efficiency. They allow companies to visualize organizational structures, market dynamics, and financial projections. One frequently employed model is the SWOT analysis, which identifies Strengths, Weaknesses, Opportunities, and Threats related to a business venture. This model offers a straightforward method for evaluating internal and external factors, aiding in informed decision-making.
Consider a startup aiming to penetrate a competitive market. By applying the Business Model Canvas, entrepreneurs can lay out crucial elements such as value propositions, customer segments, and revenue streams. This model not only facilitates clarity but also enhances communication among stakeholders, ensuring everyone is on the same page. In addition, scenario planning models help businesses prepare for uncertainties by forecasting the impact of various economic conditions, guiding them through potential pitfalls.
Models in Economics
In economics, models serve as blueprints for understanding complex systems and predicting behaviors. They support the formation of policies and help analyze the interrelationships between variables. For example, the Keynesian model emphasizes the role of aggregate demand in economic performance, influencing fiscal policy decisions.
Econometric models are extensively used to quantify relationships among economic indicators. They help economists to substantiate claims and forecast trends with statistical rigor. However, reliance on these models also requires caution; oversimplification can lead to misguided policies. Hence, models in economics must be constantly validated against real-world outcomes to maintain their relevance and accuracy.
Educational Models
In education, models assist in structuring curriculum design and instructional methods. The Constructivist model, for instance, posits that learners construct knowledge through experiences and reflections. This shifts the focus from traditional rote learning to a more interactive and engaging approach.
Models such as Bloom’s Taxonomy play a crucial role in developing educational objectives and assessments. They provide educators with a framework to categorize learning objectives, fostering a systematic approach to curriculum development. Implementing these models not only enhances teaching efficacy but also promotes a deeper understanding among students, catering to diverse learning styles and paces.
Models in Environmental Science
Environmental science heavily relies on models to assess ecosystems, predict climate changes, and develop sustainable practices. For instance, the IPCC climate models simulate climatic conditions by integrating data from various sources to forecast global warming impacts. This helps policymakers understand potential scenarios and encourages the adoption of proactive measures.
Moreover, ecological models, such as Lotka-Volterra equations, describe predator-prey relationships, aiding in biodiversity conservation efforts. These models not only illuminate complex interactions within ecosystems but also guide practical interventions aimed at preserving our planet's health.
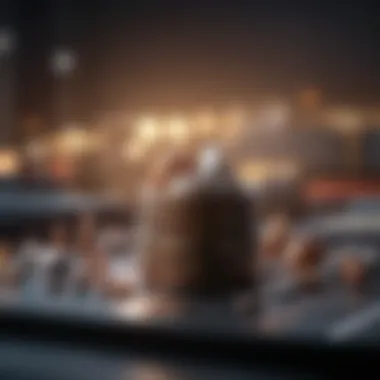
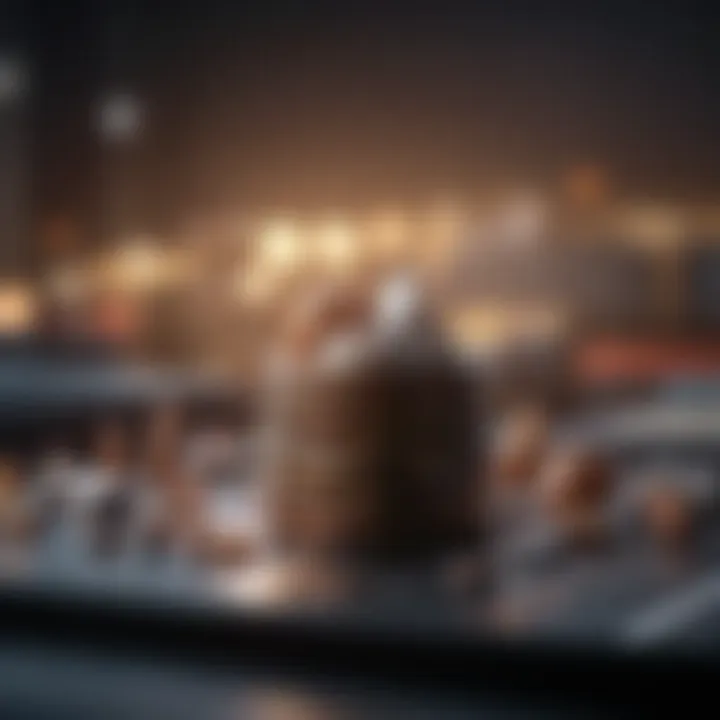
Healthcare Models
In healthcare, models are indispensable for understanding patient flow, disease progression, and treatment outcomes. The Chronic Care Model emphasizes an organized approach to patient care, enhancing the management of chronic diseases through coordinated efforts among healthcare providers.
Another significant model is the Health Belief Model, which explains health behaviors and attitudes. It helps in designing effective health interventions by understanding individuals’ perceptions of risk and benefits. By applying these models, healthcare professionals can tailor strategies that resonate with patients' needs, ultimately improving healthcare outcomes and quality of life.
"Models are not just tools; they are the lenses through which we view the world, shaping our understanding and enabling us to navigate challenges across various fields."
In summary, the applications of models in different disciplines underscore their importance as adaptable tools. They bring clarity to complex concepts, guide strategic decision-making, and foster a deeper understanding of multifaceted systems. The seamless integration of models in various fields emphasizes the need for ongoing development and validation processes to ensure their robust applicability and validity.
Model Development Process
The process of developing a model is fundamental to translating our understanding of complex systems into manageable representations. This section highlights various key elements inherent in the model development process, shedding light on its intrinsic value and significance. Engaging in this process not only structures our approach but also optimizes the applicability of models across different domains.
Identifying the Purpose
When venturing into model development, the first step is pinpointing its purpose. A model crafted without a clear intention is like setting sail without a compass. The purpose guides the direction of every subsequent step. It could range from explaining a theoretical concept to predicting outcomes in applied settings. Here, key questions emerge: What problem am I trying to solve? Who is the target audience for this model?
Identifying the purpose involves a thorough analysis of the context in which the model will be employed. Whether in economics aiming to forecast market trends or in healthcare to evaluate patient outcomes, understanding the functionality of your model lays the groundwork for its success.
Data Collection and Analysis
Once the purpose is clarified, the next critical step is data collection and analysis. This is where raw information is gathered and processed into a format suitable for model creation. Accurate data serves as the backbone of effective models. Without it, the foundations remain shaky, risking unreliable results.
Data can be collected from various sources, both quantitative and qualitative. Examples of sources might include surveys, experiments, historical records, and current statistics. It's imperative here to consider not only the volume of data but also its relevance and reliability. After collection, analysis using quantitative methods like regression analysis or qualitative assessments can yield insights that inform model parameters and dynamics.
- Data should be:
- Relevant
- Recent
- Reliable
By piecing together this data, one begins to see patterns and anomalies, paving the way to create an effective model that reflects reality more accurately.
Validation and Testing
In the final stages of model development, validation and testing become vital to ascertain the model's reliability. A model must not only be accurate but also robust against different scenarios. This step acts as a reality check, allowing developers to scrutinize the model under various conditions.
Validation involves comparing model outcomes with real-world data to assess accuracy. If discrepancies arise, it necessitates revisiting earlier stages, refining either the data collection approach or the assumptions underpinning the model. Testing could also lead to the identification of potential overfitting or underfitting issues, ensuring that the model captures the essence of the problem without being too complex or overly simplistic.
"Validation is not just a box to tick; it's a critical evaluation that ensures the model mirrors the reality it's meant to represent."
Incorporating feedback from initial testing phases directs the refinement of the model, enhancing its predictive power while ensuring its application across various scenarios.
Challenges in Modeling
When we talk about models, we’re diving headfirst into a sea of complexities. Models can serve as our anchors, helping us make sense of the turbulent waters of data and theories. However, the path of modeling is not without its hurdles. In this section, we’ll explore common challenges that can pop up during the modeling process, including complexities involved and the risks of overfitting or underfitting.
Complexity and Accuracy
Let’s start with the multifaceted nature of complexity, which looms large when developing a model. Modeling often involves synthesizing large amounts of data, which in itself can be daunting. When data is intricate and varied, the task of creating a model that accurately reflects reality becomes a puzzle. It’s crucial to strike a balance; on one hand, a model must be detailed enough to capture essential features of the data, while on the other hand, it shouldn’t be so intricate that it becomes unwieldy or hard to understand.
Take financial modeling, for example. Analysts often sift through vast datasets to create projections. Inaccurate data input or neglected variables can lead to erroneous conclusions, potentially causing substantial financial losses. Models are designed to offer clarity, but if they become too complex, they risk losing their efficacy.
Accurate models provide the foundation for informed decision-making, and achieving accuracy is not just about the model's technical prowess; it also hinges on the user’s understanding and interpretation. This is where skill comes into play. Even the most sophisticated model can fall flat if the user misinterprets its outputs.
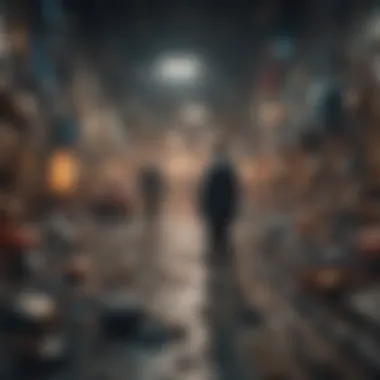
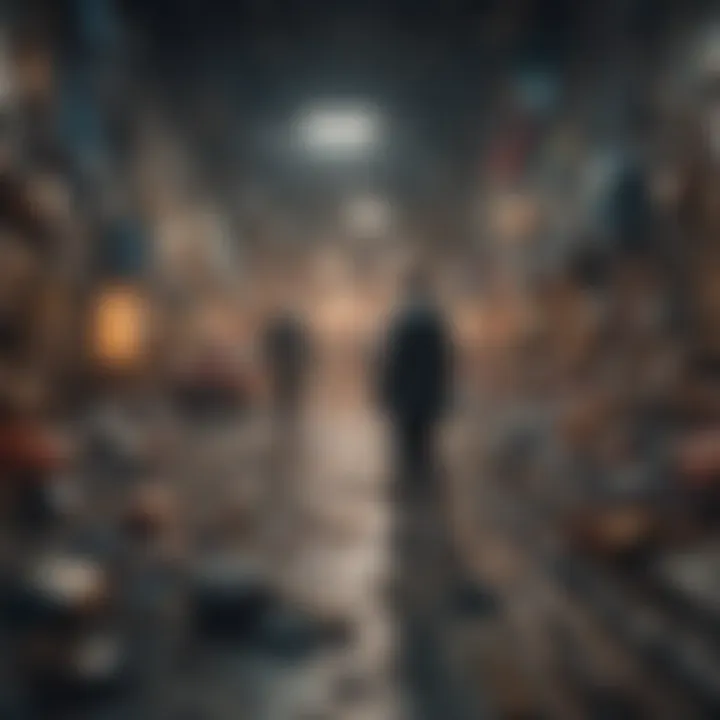
"A model is only as good as its data—and the data is only as good as the person who interprets it."
Overfitting and Underfitting
Next, we have the terms overfitting and underfitting, which essentially represent two sides of the same coin. Overfitting happens when a model learns the noise in the training data instead of the actual signal. Picture it like this: you’re studying for a test by memorizing answers, but the questions on the exam are different. You might get high accuracy on the practice tests but crash badly when it counts.
On the contrary, underfitting takes place when a model is too simple to capture the underlying trend of the data. Think of a poorly drawn map that lacks important landmarks—it may get you somewhere, but not necessarily where you need to go. Finding that sweet spot in between is what model developers strive for.
- Signs of Overfitting:
- Signs of Underfitting:
- High accuracy on training data but poor performance on new data.
- Complex models with too many parameters relative to the dataset.
- Consistently poor performance on training and test datasets.
- Simple models that do not capture significant trends or features.
As you can see, overcoming these challenges requires both technical skills and nuanced understanding of the underlying subject matter. This balancing act is not for the faint of heart but is integral in producing reliable models that can yield meaningful insights.
In the ever-evolving landscape of modeling, understanding these challenges will equip us better to handle the complexities and deliver results that matter.
Future Directions in Modeling
As we stride into a landscape shaped increasingly by rapid technological growth and ever-evolving scientific inquiries, it becomes crucial to focus on the future directions in modeling. This section emphasizes how emerging technologies and interdisciplinary approaches are likely to steer the development of models in diverse fields, ensuring that they remain relevant and productive in addressing complex issues.
Emerging Technologies
The roots of future modeling possibilities lie in the advent of emerging technologies. We stand on the brink of a technological revolution that is capable of reshaping how models are developed and utilized. For example, advancements in artificial intelligence and machine learning have introduced new methods for creating more accurate and reliable models. These technologies help analysts sift through massive datasets, pulling out trends and much needed insights that were previously obscured by information overload.
Here are several noteworthy technologies that are making waves:
- Artificial Intelligence (AI): As AI systems learn from data patterns, they can fine-tune models for predictions more adaptively than traditional methods, which tend to be static.
- Quantum Computing: Quantum computers offer unparalleled computational power, enabling the simulation of complex systems in ways classic computers never could.
- Blockchain Technology: Alongside its financial applications, blockchain provides a transparent framework for ensuring data integrity, improving the reliability of data used in modeling processes.
Investing in these technologies can offer organizations a competitive edge. The key benefit is the potential for increased accuracy and efficiency in decision-making scenarios, where speed is often as critical as precision. The challenge, however, lies in integrating these technologies into existing frameworks without overwhelming the foundational principles that models are built upon.
Interdisciplinary Approaches
An equally important direction for future modeling is the adoption of interdisciplinary approaches. As problems in today’s world become increasingly multifaceted, relying solely on one discipline can lead to a narrow perspective. Thus, blending knowledge and methods from various fields can enrich modeling practices.
Consider the following:
- Collaboration Between Sectors: The healthcare model that incorporates insights from engineering, computing, and economics. This hybrid model can provide insights into systemic inefficiencies, potentially reducing costs while improving patient outcomes.
- Integration of Social Sciences: Bringing psychology and sociology into environmental models aids in predicting human behavior in response to climate change, thereby making policies more effective.
- Cross-domain Data Sharing: Facilitating data exchange between academia, industry, and government creates more comprehensive models that reflect real-world complexities.
An interdisciplinary approach can offer an inclusive view, enabling the creation of models that are not only well-rounded but also adaptable to changing conditions. Nevertheless, fostering such collaborations requires overcoming barriers like communication gaps and differing terminologies between fields.
“In the realm of complex problems, no single field holds all the answers; it is through collective insights that we illuminate the darkest corners of uncertainty.”
In summary, the future of modeling is poised for an exhilarating evolution, driven by technological advances and collaborative efforts across disciplines. As we embrace these avenues, we position ourselves to better tackle the uncertainties of tomorrow.
Finale
The conclusion of this extensive exploration into the concept of models is not merely a wrap-up; it reflects the essence of understanding their value across various fields. Models serve as the backbone of theoretical frameworks, empirical research, and practical applications. By dissecting the traits and functionalities of different types of models, we have been able to appreciate their roles in simplifying complex realities into digestible forms. This simplification not only aids in decision-making but also fosters innovation by allowing for simulations and predictions that were otherwise unimaginable.
Summary of Key Points
- Definition and Characteristics: A model is a representation of reality, crafted to help individuals comprehend and manipulate the world around them. Whether in business, science, or social sciences, each model has unique characteristics that define its effectiveness and relevance.
- Diversity of Models: We've classified models into several categories: physical, mathematical, conceptual, simulation, and statistical, each providing distinct advantages tailored to specific needs.
- Applications: The concrete application of models spans various disciplines, from driving economic strategies in business to enhancing educational outcomes and advancing scientific discoveries. The practical implications of models highlight their critical importance in our daily lives.
- Development Process: Understanding how models are developed, from identifying a purpose to validation and testing, reveals the intricacies involved in their creation and implementation.
- Challenges and Future Directions: While models are powerful tools, they come with challenges such as complexity and the risk of oversimplification or misrepresentation. Future directions of modeling point towards greater technological integration and interdisciplinary collaboration, paving the way for more innovative solutions in understanding complex systems.
Implications for Future Research
The insights gleaned from our investigation into models indicate substantial implications for future research. As fields continue to evolve, the dynamic nature of modeling illustrates a need for adaptability. Here are a few key considerations:
- Interdisciplinary Collaboration: There’s a growing opportunity to fuse insights from multiple disciplines to create more robust models that can better analyze and predict outcomes within the interconnected realms of economy, environment, and social behavior.
- Adaptation of Emerging Technologies: The advent of AI and machine learning can enhance modeling capabilities, allowing for more extensive data sets and novel predictions. Future research may focus on how these technologies can be harnessed effectively without losing sight of theoretical foundations.
- Ethical Considerations: As models influence real-world implications, the ethical dimensions of modeling need attention. Understanding the limitations and biases present in models is critical to prevent misapplications that can have significant social consequences.
In summary, models are indispensable instruments across various sectors. Their understanding and development will continue to significantly impact how we approach and solve complex problems in the future.