Leveraging Predictive Analytics for Fraud Prevention
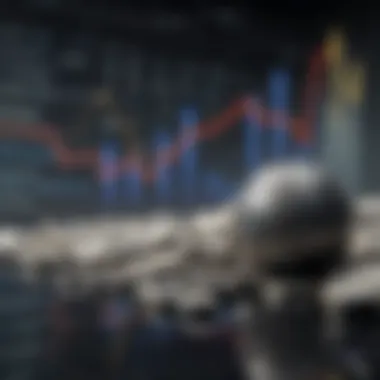
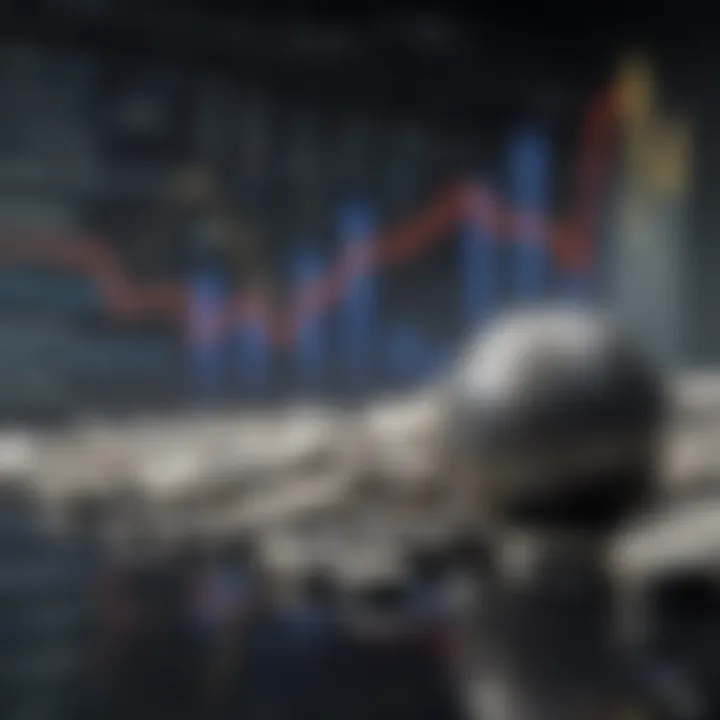
Intro
In the expanding world of finance, the increasing sophistication of fraud schemes poses a significant threat to integrity and security. There's a constant tug-of-war between those on the lookout for loopholes and the defenders who aim to keep the financial system intact. Harnessing predictive analytics is becoming an increasingly vital weapon in this battle, providing a means to anticipate and mitigate fraudulent activity before it can escalate.
Predictive analytics employs advanced statistical techniques and machine learning algorithms to analyze historical data, identify patterns, and generate insights that can forecast future outcomes. When applied to fraud detection, it helps institutions uncover suspicious behavior and anomalous transactions swiftly, thereby reducing losses and maintaining trust in financial markets.
As we delve into this narrative, the aim is to shed light on the multilayered aspects of predictive analytics in fraud detection - from core concepts and important terminologies to practical investment strategies. Let's explore this world where data meets finance, encapsulating the methodologies and implications of effectively leveraging predictive analytics.
Prolusion to Predictive Analytics
Predictive analytics has emerged as a powerful tool in various fields, especially in fraud detection. This approach leverages historical data and statistical techniques to make informed predictions about future events. For organizations aiming to combat fraud, grasping the basics of predictive analytics is not just beneficial but essential. It allows businesses to proactively identify and mitigate risks, thus protecting assets and reducing potential losses.
Definition and Overview
Predictive analytics refers to the branch of advanced analytics that uses both historical and current data to forecast future outcomes. At its core, it encompasses a range of techniques, from statistical modeling to machine learning. By analyzing patterns and trends in data, organizations can identify potential fraudulent activities before they escalate. For example, a bank might utilize predictive analytics to analyze customer transaction data, identifying unusual patterns indicative of fraud.
An essential aspect of predictive analytics is its reliance on data. The more accurate and comprehensive the data available, the better the predictive model. This capability to sift through large volumes of data to find actionable insights makes predictive analytics invaluable in fraud detection settings.
Historical Context
The roots of predictive analytics can be traced back to statistical forecasting methods that have been in use for decades. However, with the advent of big data and improved computing power, its applications have expanded significantly. Initially, businesses relied on basic regression models, but as technology evolved, more sophisticated techniques came into play.
In the late 20th century, organizations started adopting machine learning algorithms that could learn from new data patterns over time. This was crucial in fraud detection as it allowed systems to adapt and improve their predictive capabilities continuously. The increasing sophistication of fraud tactics meant that static approaches were insufficient. Now, predictive analytics stands at the forefront, offering an adaptive strategy to combat evolving fraud schemes effectively.
The Role of Data in Predictive Analytics
Data plays a pivotal role in predictive analytics. Without it, predictions would be mere guesses. High-quality, clean data is the lifeblood of any predictive model. In fraud detection, various data types are harnessedâfrom transaction records and user behavior online to demographic information.
"In today's data-driven world, the ability to harness data effectively can mean the difference between staying one step ahead of fraudsters or falling victim to their schemes."
Moreover, data integration is crucial. By combining datasets from various sourcesâsuch as financial records, social media activity, and even geolocation dataâorganizations can build a more comprehensive view of potential risks, enhancing the accuracy of predictions. The correct and judicious use of such data not only unearths existing fraud but also helps in anticipating future threats, streamlining efforts in safeguarding financial integrity.
Understanding Fraud Detection
Fraud detection is a critical area in various sectors, especially finance, e-commerce, and healthcare. Understanding how fraud occurs and the nuances of detection systems is fundamental for stakeholders trying to mitigate risks and protect assets.
Fraud, in its simplest form, refers to any deliberate act that results in financial or personal gain at another's expense. With the rapid growth of technology and online transactions, fraudulent activities have evolved too. This is not just about identifying bad actors but also about ensuring the integrity of financial systems. Having a solid grasp of the intricacies involved in fraud detection can inform better decision-making and risk assessment.
Types of Fraud
Fraud takes many guises, and distinguishing among the various types helps in crafting pointed strategies. Here are some common types:
- Identity Theft: Unauthorized use of someone else's personal data to gain financial benefits.
- Credit Card Fraud: Making unauthorized transactions using stolen card information.
- Insurance Fraud: Falsifying claims to gain undeserved benefits.
- Health Care Fraud: Billing for services that were never rendered or charging for unnecessary medical procedures.
These instances underline the necessity for robust detection systems, as understanding the type of fraud is paramount in developing an effective response.
Conventional vs. Modern Approaches
Conventional methods of fraud detection typically rely on rule-based systems. These systems use static criteria to flag problematic transactions. For instance, if a transaction exceeds a certain credit limit, itâs automatically flagged for further review. While useful, this method has several limitations:
- High False Positives: Many legitimate transactions get flagged, causing unnecessary customer frustration.
- Rigid Framework: A lack of adaptability to new fraud tactics can leave loopholes unaddressed.
- Labor Intensive: Manual review of flagged cases can drain resources.
In contrast, modern approaches employ predictive analytics and machine learning. They analyze vast datasets to identify trends and anomalies that traditional methods might overlook. By analyzing patterns, these models adapt over time, learning to recognize even the slightest changes in behavior. This results in:
- Reduced False Positives: Transactions are scrutinized with greater accuracy, freeing up investigative resources.
- Dynamic Learning: As fraudsters adapt, so do the models, making them more effective.
- Proactive Strategies: Prediction allows for identifying potential fraudulent behaviors before a crime takes place.
Challenges in Traditional Fraud Detection
While traditional methods have their place, they come with challenges that can hinder the overall effectiveness of fraud detection. Consider the following:
- Data Silos: Often, organizations have fragmented data systems that don't communicate, leading to incomplete information for fraud detection.
- Resource Constraints: The manpower needed for manual reviews can often be in short supply, leading to backlogs.
- Stagnation: A failure to update detection criteria can leave organizations vulnerable as fraud tactics evolve rapidly.
For companies to remain vigilant against fraud, understanding these challenges is essential. Acknowledging the drawbacks of conventional systems only highlights the need for the integration of advanced predictive analytics into future strategies. This not only enhances efficiency but also bolsters the overarching goal of protecting individuals and institutions from fraud.
The Intersection of Predictive Analytics and Fraud Detection
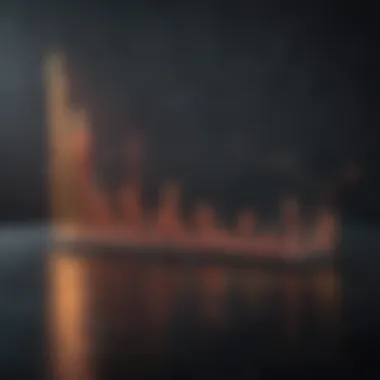
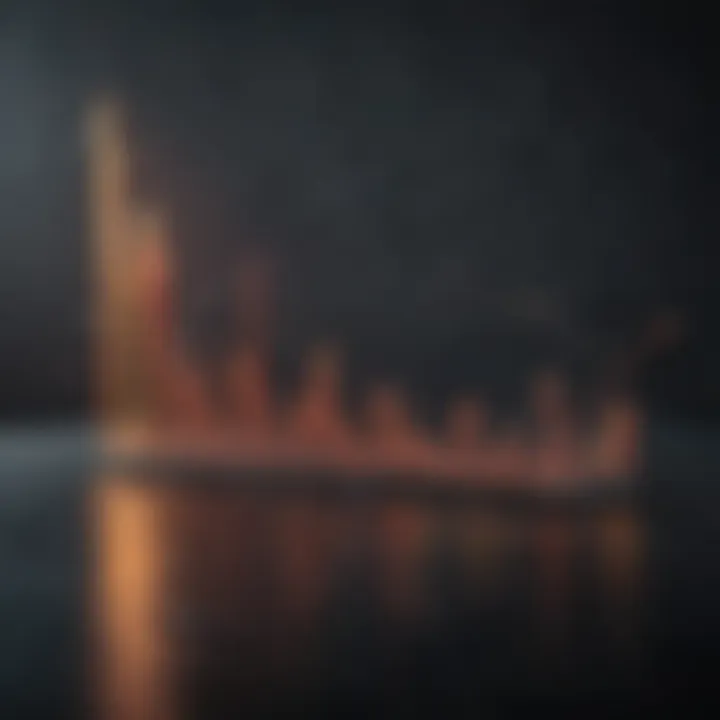
Predictive analytics holds immense importance in the realm of fraud detection, mainly due to its ability to efficiently process vast amounts of data and highlight suspicious activities before they escalate. This intersection showcases a promising partnership where data-driven insights become a shield against fraudulent behavior. Organizations can harness the power of predictive analytics to not only detect fraud early but also design preventive measures tailored to their unique operational risks. This approach transforms reactive strategies into proactive defenses, ultimately enhancing both operational efficiency and customer trust.
How Predictive Analytics Enhances Fraud Detection.
Predictive analytics enhances fraud detection in several key ways. Firstly, it leverages historical data to recognize patterns that may indicate fraudulent transactions. By analyzing previous incidents of fraud, companies can develop models that predict potential breaches based on the behaviors and characteristics typical of past offenders. This aspect allows organizations to assess real-time transactions against these models, flagging anything out of the ordinary.
Moreover, the capability of predictive analytics to sift through large data sets means it can uncover subtle, yet significant abnormalities that human analysts might overlook.
It also supports improved decision-making through:
- Real-time monitoring: Organizations can conduct ongoing assessments, making it possible to react instantaneously compared to weekly or monthly reviews.
- Resource allocation: By focusing on high-risk transactions, firms can channel their resources more effectively to areas needing the most scrutiny.
- Enhanced accuracy: Reducing false positives increases operational efficiency and ensures that genuine customers aren't unnecessarily flagged.
Thus, the integration of predictive analytics not only boosts fraud detection capabilities but also cultivates a system that learns and evolves with timeâ refining itself based on ongoing incidents.
Key Techniques Used in Predictive Models
The efficacy of predictive analytics in combating fraud is significantly heightened through various techniques. Each technique contributes a unique lens through which data is analyzed:
- Regression Analysis: This technique helps identify relationships among variables that typically influence fraudulent behavior. By understanding these relationships, organizations can predict risks based on certain transactional attributes.
- Classification Algorithms: Utilizing machine learning, classification algorithms categorize transactions into 'fraud' or 'non-fraud' based on the patterns derived from historical data.
- Anomaly Detection Models: These models signal abnormalities when new data diverges from the established norms, catching unusual transactions that may suggest fraud.
Incorporating a mix of these techniques allows companies to adopt a comprehensive approach, ensuring that fraud detection efforts are multifaceted and adaptable.
The Significance of Machine Learning
Machine learning revolutionizes the landscape of predictive analytics and fraud detection. It does this by
- Automating Processes: Machine learning algorithms learn from the data over time, automating many aspects of monitoring and detection that used to require extensive human input.
- Improving Predictions: As algorithms are exposed to more data, their forecasting accuracy enhances, reducing rates of false positives while retaining a keen eye on potential fraud.
- Adapting to New Threats: The rapidly evolving nature of fraud demands adaptability. Machine learning allows models to adjust in real time as new fraud tactics emerge, ensuring organizations stay a step ahead.
In summary, the intersection of predictive analytics and fraud detection unfolds a multifaceted landscape rich with opportunities for organizations aiming to curb fraudulent activities. Not only does it afford a proactive stance, but it imbues systems with the ability to evolve, learn, and ultimately safeguard financial integrity.
Predictive Analytics Techniques in Depth
Predictive analytics stands as a cornerstone in the fight against fraud, providing a structured approach to analyze vast datasets. Understanding the techniques involved is essential for creating effective models that can anticipate fraudulent behavior before it leads to substantial losses. By honing in on specific methodologies, businesses can tailor their strategies, directly impacting their bottom lines. Each technique offers unique insights, which can be leveraged to enhance fraud detection measures effectively.
Regression Analysis
Regression analysis serves a pivotal role in predictive analytics, particularly in estimating relationships among variables. By using historical data, businesses can uncover patterns that might suggest fraudulent activity. For example, if past data indicates that certain spending behaviors correlate strongly with fraudulent claims, businesses can use this correlation to flag similar future transactions.
There are several types of regression analysis, including linear and logistic regression. In the context of fraud detection:
- Linear Regression can be beneficial for predicting continuous outcomes, like the amount of a transaction.
- Logistic Regression is often utilized to predict categorical outcomes, such as whether a transaction is fraudulent or not.
Effective application of regression analysis requires accurate data segmentation to ensure the model's parameters are both reliable and relevant. This technique not only enhances precision in identifying fraudulent behavior but also aids in risk scoring customer profiles.
Classification Algorithms
Classification algorithms are another integral aspect of predictive analytics. They categorize data into predefined classes, which can be immensely helpful in fraud detection tasks. Trained on labeled datasets, these algorithms learn patterns from the data and can classify new, unseen transactions accordingly.
Some common classification techniques include:
- Decision Trees â a simple yet powerful method that partitions data based on decision rules.
- Random Forests â an ensemble learning method that improves classification accuracy by combining multiple decision trees.
- Support Vector Machines (SVM) â effective for high-dimensional spaces, particularly useful when fraud patterns are less obvious.
The strength of these algorithms lies in their ability to adapt and evolve with new data inputs. As they learn from ongoing transactions, they can continue refining their categorizations, making them crucial for daily operations in sectors vulnerable to fraud.
Anomaly Detection Models
Anomaly detection models focus on identifying rare items or events that deviate from an expected pattern. In fraud detection, these anomalies can signal potential fraudulent activity. Recognizing what is "normal" helps in flagging unusual behaviors that warrant further investigation.
Techniques for anomaly detection include:
- Statistical methods â using Z-scores or other statistical indicators to identify outliers.
- Machine Learning approaches â such as clustering methods which group data points, helping to identify those that don't fit well within any group.
Anomaly detection is particularly valuable in real-time fraud detection systems, as it can swiftly highlight transactions that seem suspicious without a substantial need for historical data, allowing organizations to react promptly.
Overall, these predictive analytics techniques gear organizations to harness the power of data in the fight against fraud. Knowledge of each method enhances understanding, leading to smarter and more strategic decisions, ultimately ensuring better financial security across various sectors.
Case Studies of Predictive Analytics in Action
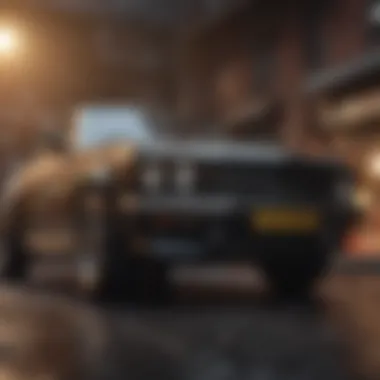
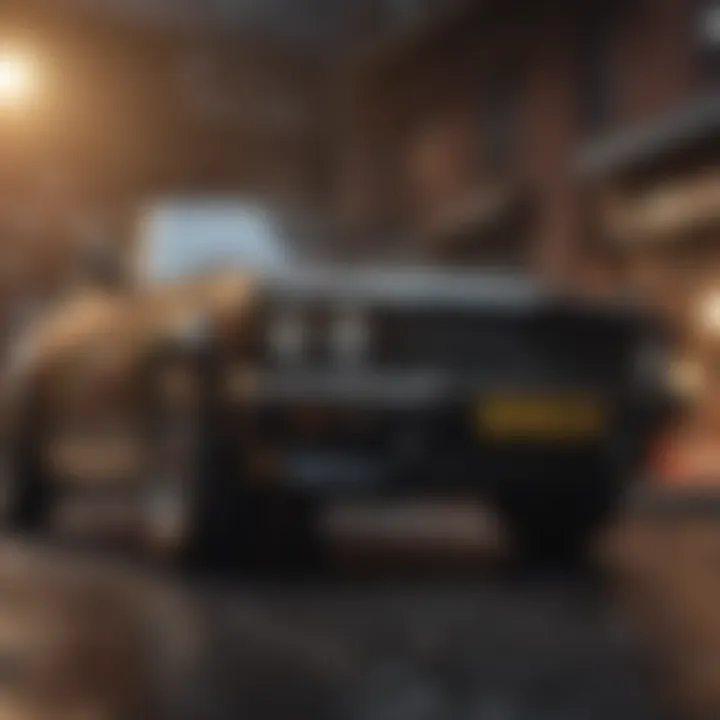
Diving into the practicality of predictive analytics in preventing fraud provides a well-rounded view of its immediate benefits and far-reaching implications. Case studies offer a window into the real-world application of these techniques, showcasing how organizations across sectors have harnessed them to tackle fraud effectively. Understanding these applications can guide other entities in not only recognizing potential fraud risks but also in proactively mitigating them, thus safeguarding their resources and reputation.
Financial Sector Applications
Within the financial sector, the stakes are often high, making the application of predictive analytics immensely valuable. Banks and financial institutions leverage data-driven strategies to predict customer behavior and detect anomalies that suggest fraudulent activity. For instance, institutions like JP Morgan Chase use algorithms that analyze transaction patterns in real time. When unusual spikes in spending or discrepancies in account activity are detected, alerts are triggered for further investigation.
Such predictive tools not only enhance fraud detection accuracy but also improve customer satisfaction by minimizing false positives. When a system can differentiate between an actual fraudulent transaction and a legitimate one â perhaps by analyzing spending patterns over time â it allows for smoother customer experiences while decreasing operational costs related to fraud investigation.
Key Benefits in Finance:
- Enhanced real-time alerting on unusual activities.
- Increased accuracy in fraud detection, leading to reduced losses.
- Improved customer trust through seamless transaction handling.
Healthcare Fraud Detection
Healthcare is another critical domain where predictive analytics emerges as a game-changer. Fraud in this area is multifaceted, involving everything from billing for services not rendered to providing unnecessary treatments. Organizations such as the Centers for Medicare & Medicaid Services (CMS) have implemented predictive models to identify suspicious billing patterns.
One notable case involved analyzing physician claim data to uncover providers billing for excessive services compared to peers in similar specialties. By adopting a predictive approach that incorporated various data points, the CMS was able to halt numerous fraudulent practices, saving millions for taxpayers. This proactive stance not only deters future fraud but also promotes ethical practices in healthcare delivery.
Significant Insights in Healthcare:
- Continuous monitoring of claims for anomaly detection.
- Reduction in fraudulent billing through targeted investigations.
- Promotion of ethical standards within healthcare systems.
E-commerce and Online Fraud Prevention
In the fast-paced world of e-commerce, the battle against fraud is relentless. Online merchants face threats ranging from stolen credit card information to sophisticated phishing schemes. Companies like PayPal have turned to predictive analytics to enhance their fraud detection capabilities. They utilize machine learning algorithms that evaluate the risk of a transaction based on historical data and behavioral analytics.
By assessing factors such as purchase frequency, user geolocation, and payment methods, these systems can flag high-risk transactions. This not only protects customers but also enables businesses to maintain their reputation by handling potential fraud scenarios swiftly. The integration of predictive analytics here allows for a more relaxed customer experience while providing robust security measures.
E-commerce Aspects to Consider:
- Machine learning algorithms for real-time risk assessment.
- Enhanced customer protection leading to increased loyalty.
- Streamlined operations with automated fraud detection systems.
"These case studies demonstrate that predictive analytics is not merely a theoretical concept; it's a vital tool that drives action and ensures security in fraud prevention."
By analyzing the successes and strategies of various sectors employing predictive analytics, organizations can benchmark their own practices. These examples serve to illuminate the path forward for businesses aiming to integrate similar methodologies, ultimately reinforcing their defenses against fraud.
Ethical Considerations and Data Privacy
In the age of digital transformation, where every cherry-picked piece of data can hold monumental significance, the intersection of predictive analytics and fraud detection brings about a goldmine of possibilities. However, with this wealth comes an undeniable responsibility. The ethical considerations and data privacy measures surrounding the application of predictive analytics are paramount. Without adherence to these principles, we risk not only the integrity of the analytical models but the trust of the individuals and entities being analyzed.
Managing Data Responsibly
When we talk about managing data, weâre not merely tossing terms around; weâre diving into a highly sensitive realm. Data integrity is severely tested when predictive analytics is involved, especially when personal or financial information is in the mix. Putting data to good use while ensuring responsible management involves some fundamental practices:
- Data Minimization: Collect only what is necessary for a specific purpose. Too much information can raise red flags and impede ethical practices.
- Anonymization Techniques: Whenever possible, tighten up the security of personal data by removing identifiable information. This is a good practice to mitigate risks while analyzing ample datasets.
- Transparency in Data Use: Users should always be informed about how their data is being used. When companies step up and share this information, it fosters trust, laying the groundwork for responsible data handling.
The emphasis on responsible data management not only protects consumers but also helps organizations steer clear of potential legal pitfalls.
Compliance with Regulations
Everyone knows that playing by the rules is necessary, and itâs especially true in data handling. With a labyrinth of regulations surrounding data usageâfrom GDPR in the EU to the CCPA in Californiaâcompanies are required to stay up-to-date with legal requirements. This becomes all the more pressing when algorithms start cranking out predictive analyses based on sensitive data. Following robust compliance pathways involves:
- Staying Informed: Regularly check updates in policies that relate to data privacy and consumer rights. A misstep today can lead to enormous fines tomorrow.
- Establishing Protocols: Organizations should develop clear protocols to comply with regulations related to data collection, storage, and usage. Document everything to maintain a transparent flow of information.
- Regular Audits: Conduct periodic reviews of data practices to ensure adherence to established protocols. An internal audit every so often can help nip issues in the bud before they spiral out of control.
Observing compliance isnât just about avoiding penalties; itâs about enhancing the credibility of predictive analytics as a trustworthy tool in fraud detection.
Bias in Predictive Models
Ah, bias, the elephant in the room that nobody wants to talk about but is always lurking in the shadows. Predictive models are built on data, and if the underlying datasets exhibit biases, the outcomes are bound to reflect these discrepancies. This can lead to skewed analyses that hurt rather than help. Tackling bias encompasses several key areas:
- Diverse Datasets: Itâs crucial to input diverse datasets to ensure that different perspectives are accounted for. A model trained on limited data may inaccurately predict certain behaviors or trends.
- Bias Detection Mechanisms: Integrating mechanisms that identify and address biases can significantly enhance the integrity of predictive analytics. Regularly testing models for performance across various demographics helps refine results.
- Continuous Feedback Loops: Engaging a variety of stakeholders throughout the modeling process ensures continuous scrutiny of potential biases. Their insights help fortify the modelâs reliability.
"Predictive analytics can be a double-edged sword; it's up to us to wield it wisely to glean valuable insights without compromising ethics or privacy."
In sum, the practice of ethical considerations and data privacy while harnessing predictive analytics cannot be overstated. As we further delve into the digital age, balancing innovation with responsibility will serve as the cornerstone of effective fraud detection solutions.

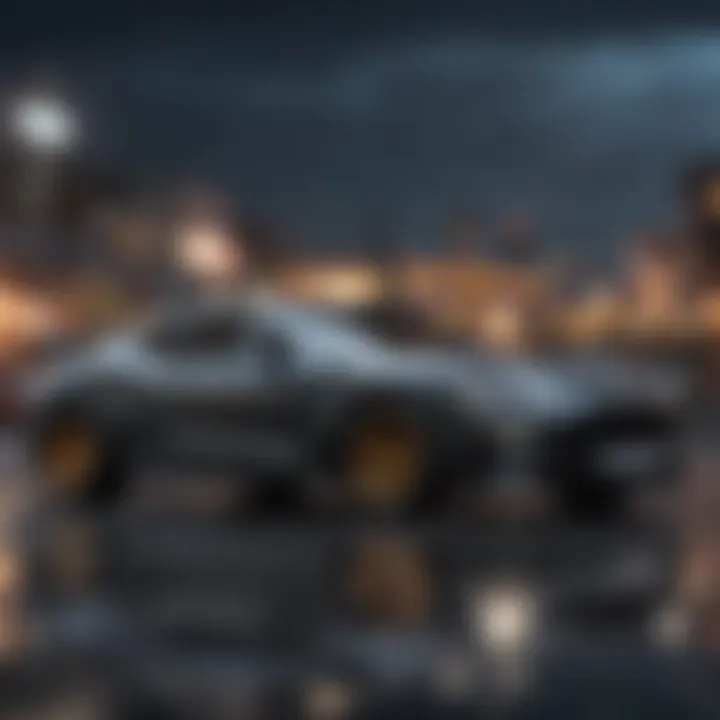
Future Trends in Predictive Analytics and Fraud Detection
The integration of predictive analytics in fraud detection is evolving rapidly, and understanding the trends shaping this field is essential for those engaged in safeguarding financial integrity. Innovations in technology and methodologies are not just shaping how fraud is detected and dealt with but also offering insights that can preemptively address vulnerabilities before they become costly issues. Here, weâll delve into the future trends that promise to redefine the landscape of fraud detection.
Emerging Technologies
New tools and technologies are sprouting like weeds in a spring garden, bringing significant improvements to fraud detection capabilities. For instance, the adoption of blockchain technology has caught the eyes of many in the finance sector, mainly due to its inherent security features. With its decentralized nature, blockchain can create an immutable record of transactions, reducing the chances of fraud through sheer transparency. Other technologies making waves include cloud computing and big data analytics. With the massive amounts of data being generated daily, cloud infrastructure allows firms to analyze this data swiftly and efficiently.
Another noteworthy advancement is the increasing use of Internet of Things (IoT) devices. These gadgets provide a treasure trove of real-time data that can help detect anomalies and suspicious activities. For example, in retail settings, smart sensors can track purchasing behaviors closely enough to flag any inconsistencies that might suggest fraudulent activities.
Integration with Artificial Intelligence
Artificial intelligence stands at the forefront of transforming fraud detection strategies. The prowess of AI lies in its ability to learn from vast datasets and adapt over time. Imagine a system that scrutinizes patterns, identifies anomalies, and makes predictions with minimal human intervention. This is not merely a concept; itâs happening right now.
Machine learning algorithms can evaluate historical transaction data and user behaviors to forge predictive models. These systems can hone in on fraudulent activities with surprising accuracy, often recognizing complex fraudulent schemes that traditional methods fail to detect. Moreover, as these AI models train on more data, they become increasingly adept at distinguishing between genuine and fraudulent transactionsâessentially fine-tuning their intuition.
Natural Language Processing (NLP) also makes a significant impact. By analyzing unstructured data like customer complaints and social media posts, NLP can identify potential fraud trends and help organizations adjust their strategies proactively, rather than reactively.
The Evolution of Fraud Tactics
With the advancements in predictive analytics and detection tools comes an inevitable arms race between fraudsters and security measures. As organizations bolster their defenses, criminals are not sitting idle; they are evolving their tactics to exploit new vulnerabilities.
It's vital to recognize the shift towards cyber fraud, which leverages technology to carry out scams. Phishing, for instance, has moved beyond traditional email to sophisticated social engineering tactics, making the relationship between user trust and technology more tenuous. Digital wallets, cryptocurrencies, and peer-to-peer transactions, while beneficial, also present new avenues for fraud, necessitating advanced detection methodologies to address these challenges.
Additionally, the emergence of synthetic identity fraud is alarming. Fraudsters create fake identities using real information of innocent individuals. This tactic can slip through the cracks of conventional fraud detection systems which often rely on set patterns and indicators. Therefore, predictive analytics will need to evolve continuously to stay ahead of these cunning tactics that criminals are bound to innovate.
"The future of fraud detection will not just be reactive; it will be preventative. That's the promise of predictive analytics, an armory of tools that keep evolving along with the threats."
Challenges in Implementing Predictive Analytics
The integration of predictive analytics into fraud detection systems is not a walk in the park. While the potential benefits shine bright, several challenges can dim the enthusiasm of organizations eager to adopt these technologies. This section will explore the fundamental difficulties as well as the broader implications related to adopting predictive analytics in fraud detection.
Technical Barriers
Implementing predictive analytics can sometimes be like trying to fit a square peg into a round hole. One significant technical barrier is the quality and the format of the data being used. If organizations donât have clean, structured data, they could end up chasing shadows. Accessing real-time data that influences fraud detection models is crucial. But systems need to be robust enough to handle large volumes of data without breaking a sweat. Moreover, technical proficiency is needed. Without skilled professionals versed in data analysis and machine learning, businesses might find themselves with a shiny new tool that they canât effectively utilize.
Here are some of the technical challenges:
- Data Integration: Different departments may use various systems, leading to disjointed data that makes it hard to draw accurate conclusions.
- Model Complexity: Developing predictive models can be complicated, requiring understanding of both the domain and the modeling techniques.
- Real-Time Processing: Fraud detection requires near real-time insights, needing advanced infrastructure to analyze data as it flows in.
Organizational Resistance
Tackling organizational resistance is much like trying to steer a large ship: it takes considerable effort and can't be done in a hurry. Sometimes, stakeholders worry that predictive analytics will undermine their authority, while others may not fully understand its potential benefits. It's human nature to be skeptical, especially when change is in the air. Convincing everyone aboard about the importance of using these advanced techniques can be a slow burn, requiring patience and a well-laid out strategy.
Some common sources of resistance include:
- Fear of Job Displacement: Employees may worry that these systems will lessen the need for human oversight, potentially leading to layoffs.
- Knowledge Gaps: A lack of understanding or awareness of predictive analytics can cultivate skepticism among decision-makers.
- Cultural Barriers: Certain corporate cultures may be resistant to change, favoring traditional methods over innovative approaches.
Cost Implications
"To make an omelet, you have to break some eggs," the saying goes, and while itâs true that potential cost savings can arise from effective fraud detection, the initial setup costs can be a hard pill to swallow for many organizations. The investment in technology, training, and ongoing maintenance can mount quickly, and for smaller companies, these costs can lead to some serious second thoughts.
Consider the following financial aspects:
- Software and Tools: Quality predictive analytics software often comes with a hefty price tag. This includes licensing fees and ongoing costs for updates.
- Training and Talent Acquisition: Hiring skilled data scientists or training existing staff can take a toll on budgets, especially when the demand for talent is high.
- Operational Expenses: Integrating new technology may require additional resources, like new hardware or more robust IT support, amplifying costs further.
"While predictive analytics can lead to significant savings and enhanced fraud detection, organizations must tread carefully to balance the costs against the potential benefits."
Culmination
Summary of Insights
Throughout the article, we explored several key elements. First and foremost, predictive analytics serves as a vital tool in the financial sector, enhancing the capacity of organizations to navigate the complex landscape of fraud. Weâve seen how various data analysis techniques, such as regression analysis, classification algorithms, and anomaly detection models, each play a unique role in uncovering suspicious patterns that may indicate fraudulent behavior.
Moreover, with case studies from sectors like finance and healthcare, we illustrated real-world applications that showcase the effectiveness of these techniques in practice. Noteworthy was the discussion on challenges faced by organizations when implementing predictive analyticsâhighlighting barriers such as cost implications, technical limitations, and organizational resistance.
The Way Forward
Looking to the future, the evolution of fraud tactics will make the role of predictive analytics even more crucial. As criminals become increasingly sophisticated, organizations must evolve alongside them. Integrating advanced technologies such as artificial intelligence with predictive analytics could dramatically enhance detection capabilities, allowing for real-time insights that adapt to emerging threats.
In addition, a commitment to ethical considerations and data privacy must accompany any effort to enhance fraud detection. Organizations will need to navigate legal compliance while managing data responsibly. This becomes more salient as consumers' trust depends on knowing their data is secure and being used effectively.
In summary, the path forward involves not only adopting predictive analytics as a norm but also fostering a culture of continuous improvement and learning, ensuring that organizations are ever-ready to tackle the chronic issue of fraud head-on.