Understanding Predictive Analytics as a Service
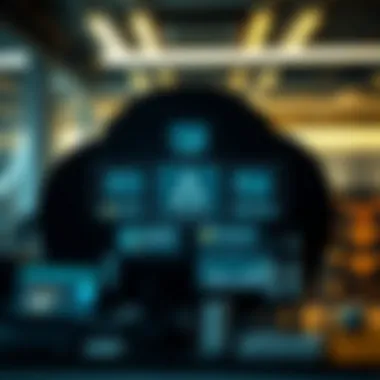
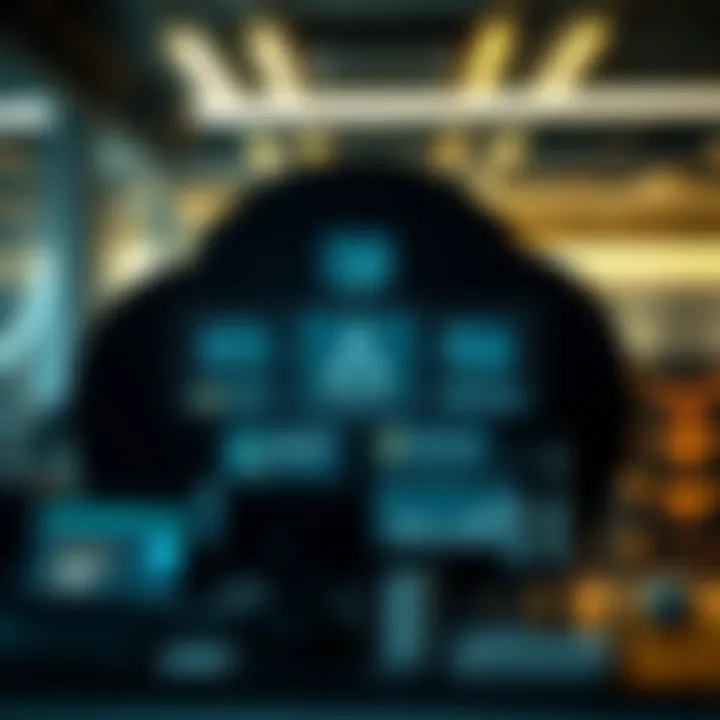
Intro
Navigating the intricate landscape of modern finance often requires a compass to guide decision-making. In this context, Predictive Analytics as a Service (PAaaS) emerges as an essential tool for cutting through the complexities of data interpretation and foresight. PAaaS combines advanced algorithms with vast amounts of data to provide actionable insights. Whether you are an individual investor looking to refine your strategies or a seasoned professional seeking to enhance operational efficiency, understanding PAaaS is crucial.
This section sets the stage for a comprehensive exploration of predictive analytics, discussing its foundational concepts, practical applications, and multifaceted benefits across various industries. By delving deep into the architecture and components of PAaaS, we will clarify what separates it from traditional analytics. Additionally, we'll touch upon the ethical considerations, challenges that accompany its integration, and the broader implications it has for the future of strategic decision-making.
Let's begin by establishing a clear understanding of the terminology and definitions associated with Projected Analytics as a Service.
Understanding Predictive Analytics
Predictive analytics is more than just a buzzword; it represents a pivotal shift in how organizations interpret data and make informed decisions. This section aims to shed light on the essence of predictive analytics and its role in today's data-driven world. Individuals and companies alike are recognizing that the ability to foresee potential outcomes based on historical and current data can be the difference between success and failure. Thus, understanding the core tenets of predictive analytics is crucial for anyone keen on navigating today’s complex business landscape.
Definition and Key Concepts
At its heart, predictive analytics employs statistical algorithms and machine learning techniques to identify the likelihood of future outcomes based on historical data. It is rooted in the premise that past behavior can predict future behavior.
Key concepts include:
- Data Mining: This involves sifting through large datasets to extract meaningful patterns. Data mining is fundamental in discovering insights and making educated predictions.
- Descriptive Analytics: Although predictive analytics focuses on future predictions, understanding what has already happened is crucial. This is where descriptive analytics steps in, helping to summarize past data for better insights.
- Predictive Modeling: Essentially the crown jewel of predictive analytics. This process uses historical data combined with algorithms to create a model that can predict future events. Various techniques like regression analysis and decision trees are often employed here.
- Machine Learning: An indispensable part of predictive analytics, machine learning algorithms enhance the accuracy of predictions by learning from data over time.
Grasping these concepts will empower financial enthusiasts to leverage analytical tools effectively, leading to more accurate forecasting and strategy formulation.
Historical Evolution
Predictive analytics has undergone significant transformations since its inception. In its nascent stages, it was primarily used in sectors like finance and marketing. In the early days, statistical methods dominated, relying heavily on simple linear regressions and basic forecasting techniques. However, as technology advanced, so too did the methods employed.
With the rise of big data, perhaps a decade ago, practitioners began integrating complex algorithms and machine learning approaches into their predictive frameworks. This evolution allowed organizations to handle vast datasets, gleaning nuanced insights that were previously unattainable. Companies began turning to this technology to anticipate customer behavior, optimize supply chains, and manage risks more effectively.
In recent years, with the advent of sophisticated cloud computing solutions, even smaller businesses have been able to harness the power of predictive analytics. They can access advanced tools without the burden of heavy upfront investments or infrastructure costs. This democratization of predictive analytics has opened up new avenues for data-driven decision-making across various industries.
"The future belongs to those who prepare for it today."
– Malcolm X
The importance of predictive analytics in understanding market trends and consumer behaviors cannot be overstated. As we continue exploring the service aspect of predictive analytics, we will unearth how organizations can capitalize on these capabilities to navigate their respective markets with confidence.
The Fundamentals of Predictive Analytics as a Service
Understanding Predictive Analytics as a Service (PAaaS) is crucial for any organization trying to stay ahead in today's competitive marketplace. This approach merges advanced data analytics with service-oriented methodology, offering businesses the tools necessary to forecast future trends. What's particularly noteworthy is how PAaaS provides access to predictive analytics capabilities without the heavy lifting that typically accompanies the setup, maintenance, and skill development.
Ultimately, organizations can enjoy a more agile response to changing market conditions, making PAaaS an invaluable asset.
Foreword to PAaaS
PAaaS represents a paradigm shift in how data analytics is delivered and consumed. Traditionally, organizations had to build their own analytics infrastructures, which required substantial time and resources. With PAaaS, businesses can tap into hosted and scalable predictive analytics solutions as a subscription service. Essentially, it's like having a data scientist in your back pocket—on-call for insights at the click of a button.
Key benefits include:
- Scalability: Easily adjusts resources according to demand.
- Cost-Effectiveness: Reduces need for upfront investment in technology.
- Access to Expertise: Valuable insights generated without needing an in-house team of data scientists.
This sort of democratization of analytics means that even smaller companies can start making data-driven decisions, leveling the playing field. With PAaaS, the barrier to entry lowers, allowing all players in the market to derive insightful conclusions without being bogged down by technical complexity.
Core Components
When dissecting PAaaS, there are several core components that come into play. Each piece serves a distinct function in weaving together the whole fabric of predictive analytics capabilities:
- Data Management: At the heart of PAaaS lies robust data management. This includes data collection, cleaning, and integration from multiple sources to form a cohesive dataset, ready for analysis.
- Modeling and Algorithms: PAaaS platforms utilize advanced machine learning and statistical models. They enable organizations to generate predictions based on historical data—be it purchasing behavior, market trends, or customer churn rates.
- User Interface: An intuitive user interface allows non-technical users to engage with the data meaningfully. Dashboards often present data trends visually, making it easier to glean insights instantaneously without requiring a deep understanding of the underlying analytics processes.
- Integration: Seamless integration with existing software ecosystems ensures that PAaaS solutions do not operate in a silo. They work in tandem with CRM, ERP, and other company systems, delivering insights wherever they can make the most impact.
- Support and Maintenance: A distinguishing feature of PAaaS is the ongoing support and updates provided by service providers. Whether it’s troubleshooting issues or rolling out the latest software updates, organizations can focus on using insights rather than managing the technical details.
By understanding these core components, businesses can better evaluate which PAaaS solution aligns best with their strategic goals. Each element plays a pivotal role in transforming raw data into actionable insights, making it imperative for organizations to choose wisely.
Comparing PAaaS with Traditional Analytics
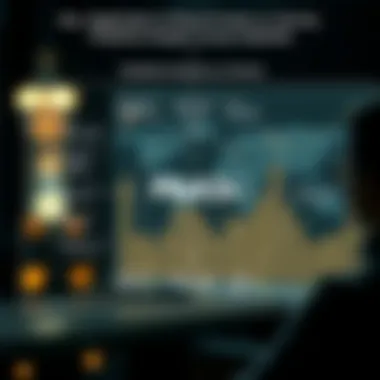
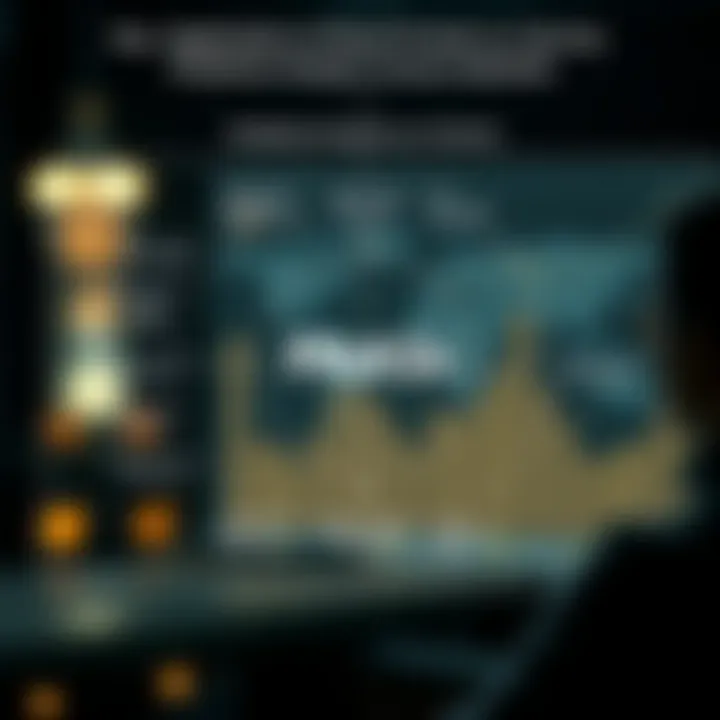
In the ever-evolving landscape of data analytics, the contrast between Predictive Analytics as a Service (PAaaS) and traditional analytics methods has become increasingly relevant. Understanding these differences is crucial, especially for financial enthusiasts and individual investors keen to leverage data for informed decision-making. By examining the unique strengths of PAaaS, stakeholders can better navigate the complexities of modern analytics and gain a competitive edge.
Differences in Approach
When we delve into the differences in approach between PAaaS and traditional analytics, it’s evident that each methodology embodies distinct philosophies. Traditional analytics often relies heavily on descriptive and diagnostic techniques. These methods focus on what has happened in the past and aim to pinpoint reasons behind those outcomes. While useful, such an approach can be limited in its predictive capabilities.
On the opposite side, PAaaS introduces a forward-thinking lens, emphasizing predictive and prescriptive analytics. This means that instead of merely reflecting on historical data, organizations can harness the power of statistical algorithms and machine learning models to make forecasts about future events. By utilizing real-time data streams, PAaaS offers a level of agility and adaptability that traditional methods simply cannot match.
This divergence in approach can be summarized as follows:
- Traditional Analytics: Focuses on what happened and why. Often uses static datasets and predefined reports.
- PAaaS: Predicts future outcomes and provides actionable recommendations. Leverages dynamic data for ongoing insights.
Advantages of PAaaS
The advantages of PAaaS are noteworthy and can significantly influence decision-making processes. Here are some key benefits to consider:
- Scalability: PAaaS platforms are inherently scalable, meaning they can easily adjust to the growing data demands of businesses. This is in stark contrast to traditional methods, which often require substantial infrastructure investments to accommodate increased data volume.
- Cost-Effectiveness: Often marketed on a subscription basis, PAaaS can lower upfront costs for organizations, enabling them to access advanced analytics without heavy initial expenditures. This is particularly beneficial for individual investors and smaller firms.
- Time Efficiency: Quick implementation and ease of integration with existing tools make PAaaS significantly faster in delivering insights. This rapid turnaround can be invaluable in the dynamic financial markets where timely information is key.
- Advanced Tools: Many PAaaS solutions come equipped with sophisticated algorithms and models that may not be accessible through traditional analytics. These advanced tools can provide a deeper understanding of complex data patterns.
- Future-Proofing: As technology evolves, the best PAaaS providers regularly update their offerings, ensuring that organizations remain equipped to tackle emerging data challenges. Traditional analytics may lag in this respect, requiring more effort to stay current.
"As businesses strive for greater efficiency and insight, adopting Predictive Analytics as a Service can be a game changer in turning data into a strategic asset."
In summary, while traditional analytics has its merits, PAaaS presents an array of benefits that can enhance the analytical capabilities of organizations and individual investors alike. This shift toward predictive methodologies marks a significant evolution in how we utilize data to inform decisions.
Applications of Predictive Analytics as a Service
Predictive Analytics as a Service (PAaaS) allows organizations to tap into powerful data analysis without the heavy lifting associated with traditional setups. The flexibility and scalability embedded in PAaaS make it a fitting choice for various industries. Diving into the applications of PAaaS can reveal how different sectors harness its capabilities to drive informed decision-making, optimize processes, and enhance overall performance.
Industry-Specific Use Cases
Across industries, predictive analytics serves as a game-changer. Below are several practical applications that highlight its profound impact:
- Healthcare: Hospitals utilize predictive models to forecast patient admissions and optimize staffing. For instance, when a particular ailment surges due to seasonal changes, hospitals can anticipate patient inflow accordingly to allocate resources effectively.
- Retail: Retailers often leverage PAaaS to analyze customer purchasing patterns. Using historical data, companies like Walmart predict inventory needs, ensuring that stocks match consumer demand while reducing overhead costs.
- Finance: Financial institutions, such as banks, employ predictive models to assess credit risk and detect fraudulent activities. By examining transaction patterns, banks can pre-emptively identify suspicious behavior, bolstering security for customers and the institution alike.
- Manufacturing: In this sector, predictive analytics supports maintenance and operational efficiency. Manufacturers use sensors and IoT devices to collect data on machinery performance, anticipating outages and streamlining repair processes before problems arise.
- Telecommunications: Companies like Verizon and AT&T apply predictive analytics to reduce churn rates. By identifying customer behavior patterns, they proactively engage users likely to leave, deploying targeted offers and improving retention.
These are just a few examples, yet they underscore the versatility of PAaaS across different sectors. Each industry adapts the tools offered by predictive analytics to fit its unique challenges and objectives.
Consumer Behavior Insights
Understanding consumer behavior is pivotal for businesses aiming to grow their market share. PAaaS can unearth trends and provide insights that drive marketing strategies. Consider the following points:
- Segmentation: By analyzing customer demographics and purchase history, businesses can segment their audience into groups, tailoring marketing strategies for each segment. A data-driven approach leads to more effective campaigns.
- Customer Journey Analysis: PAaaS allows tracking of user interactions across various touchpoints, helping companies uncover pain points in the customer journey. By resolving these friction areas, they can enhance user experiences and improve conversion rates.
- Predictive Modeling: Companies can predict future buying behaviors based on historical data. When consumers show interest in a product, predicting their likelihood to purchase can help businesses allocate marketing resources more effectively.
- Churn Prediction: Identifying factors that lead to customer churn is vital. By analyzing data with PAaaS, businesses can create models that help predict which customers might be at risk of leaving, allowing them to take preemptive actions.
"Using data wisely not only helps in enhancing customer relationships, but also boosts the bottom line for businesses."
Benefits of Implementing PAaaS
The implementation of Predictive Analytics as a Service (PAaaS) offers a plethora of advantages that are hard to ignore, especially for organizations seeking to optimize their operations. As businesses continuously navigate the complexities of modern data landscapes, the need for precise and actionable insights has never been greater. Here, we break down the key benefits into two critical areas: improved decision-making and cost efficiency.
Improved Decision-Making
In today's fast-paced market, making quick and informed decisions can set a company apart from its competitors. One of the most significant benefits of PAaaS is its ability to transform data into valuable insights, driving more accurate decision-making.
Predictive models can analyze historical data and identify trends, allowing businesses to anticipate future events—be it customer behavior, market trends, or operational hurdles. Think of it like having a crystal ball that tells you what’s coming your way. Businesses can run simulations to see the potential outcomes of various strategies without the risk involved. This not only bolsters confidence in decision-making but also enhances strategic planning.
"Data is the new oil; refine it to fuel your decision-making processes."
Furthermore, PAaaS provides tools that readily analyze raw data. For instance, platforms such as DataRobot and RapidMiner enable organizations to harness machine learning techniques efficiently. These tools can enhance predictive capabilities without the hefty investments that traditionally come with advanced analytics setups.
The speed at which organizations can adapt their strategies, based on insights gleaned from predictive analytics, can make or break their success in any given field. The agility afforded by PAaaS ensures that decision-makers can pivot quickly and act proactively rather than reactively.
Cost Efficiency
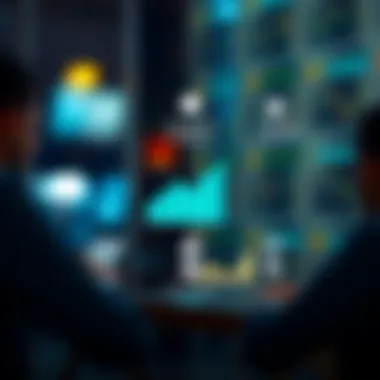
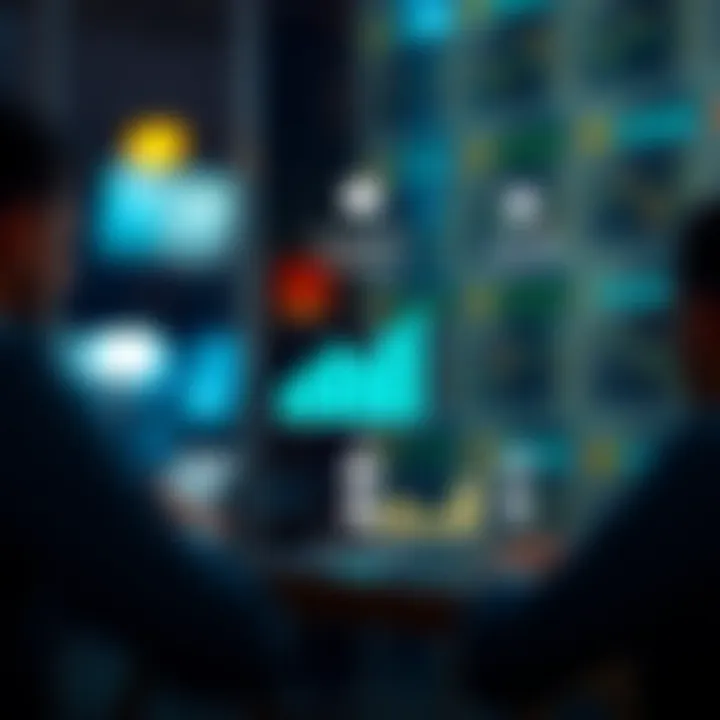
Cost efficiency is another significant factor that drives the adoption of PAaaS among organizations. Traditionally, implementing predictive analytics required substantial financial resources—considerable investments in on-premises infrastructure, software licenses, and skilled personnel. PAaaS alleviates many of these costs by providing a subscription model that allows businesses to pay for only what they use.
By leveraging third-party services, companies can avoid upfront costs associated with physical infrastructure, instead redistributing those funds into other critical areas such as innovation or customer service. This operational model not only streamlines costs but also fosters flexibility. Organizations can scale their analytics capabilities as needed, going up or down depending on their current goals and data needs.
Some key takeaways about cost efficiency in the context of PAaaS include:
- Reduced CAPEX: Lower initial investment compared to traditional analytics solutions.
- Flexible Pricing Models: Subscription services often come with tiered pricing, allowing businesses to choose what fits their needs.
- Lower Maintenance Costs: With a PAaaS provider handling system updates and maintenance, resources can focus on core business activities instead of managing complex systems.
Challenges and Considerations
Navigating the terrain of Predictive Analytics as a Service (PAaaS) isn't all sunshine and rainbows. While the potential for enhanced decision-making and efficiencies is tantalizing, certain challenges loom large. Recognizing and addressing these hurdles is essential for organizations hoping to reap the benefits of PAaaS. Here, we'll delve into two significant considerations: integration with existing systems and concerns regarding data privacy and security.
Integration with Existing Systems
Integrating PAaaS into an organization's existing technological framework can feel a bit like trying to fit a square peg in a round hole. Many businesses have legacy systems that may not interact smoothly with new predictive analytics tools. This lack of compatibility can be a nightmare, often leading to disruptions and delays.
- System Compatibility: A thorough assessment of current systems is vital before implementing PAaaS. If your existing infrastructure is outdated, you might face an uphill battle.
- Data Silos: Often, valuable information resides in separate databases. Combining these fragmented data sources into a unified analytics platform can be a monumental task. Organizations should strive for a cohesive data strategy that breaks down these silos to harness the true power of PAaaS effectively.
- Training and Adaptation: The human element can't be overlooked. Employees will need training to get comfortable with new analytics tools. Resistance to change can stall efforts, making change management crucial for successful integration.
In summary, successful integration is not just about the technology; it's also about aligning systems, breaking down data barriers, and fostering a culture of adaptability.
Data Privacy and Security Concerns
As organizations gather more data to fuel their predictive analytics, the importance of data privacy and security becomes paramount. The stakes are high; a single breach could not only endanger sensitive information but also damage a company’s reputation beyond repair.
- Compliance Issues: Regulations like GDPR and CCPA impose stringent guidelines on how personal data is collected and used. Failing to comply could result in hefty fines or legal repercussions. Understanding and implementing these regulations is not optional; it’s a necessity.
- Risk of Data Breaches: With more data being shared and stored in the cloud, the risk of cyber attacks increases. Organizations must invest in robust security measures to safeguard their predictive analytics platform. This includes encryption, secure access protocols, and regular audits to keep threats at bay.
- Trustworthiness of Data Sources: Relying on external data sources for predictive analytics necessitates vigilance. If the data lacks integrity or has been manipulated, it can lead predictive models astray, resulting in bad decisions. Organizations need to establish a strong vetting process to ensure data accuracy and reliability.
"Data privacy is not just about securing the information; it’s about fostering a culture of trust with your customers."
In closing, while the integration of PAaaS can be challenging, and the concerns over data privacy may seem daunting, being proactive and organized can help mitigate those risks. This awareness equips organizations to navigate these challenges, turning potential pitfalls into stepping stones towards successful predictive analytics endeavors.
Ethical Implications of Predictive Analytics
The influence of predictive analytics in today's digital landscape is undeniable, yet it brings forth a myriad of ethical complexities that cannot be ignored. As organizations increasingly depend on data-driven insights, understanding the ethical implications of these tools becomes paramount, especially in a world where data misuse can lead to severe consequences. This section will delve into two critical aspects of these ethical considerations: the issues of bias and discrimination and the necessity for transparency in algorithm usage.
Bias and Discrimination Issues
Predictive analytics, by its nature, relies on historical data to forecast future outcomes. However, if the data used in this predictive modeling is biased, the results will inevitably reflect those biases. For instance, if a financial institution uses historical lending data to predict creditworthiness, but that data is skewed against certain demographics, it can perpetuate existing inequalities. The implications are not just theoretical; they can significantly impact opportunities for individuals in underrepresented groups.
"Data is just a reflection of the past, and if that past is laced with biases, the analytics derived from it will only reinforce those patterns."
When considering bias in predictive analytics, it’s essential to understand how data collection methods might unintentionally favor certain groups over others. Moreover, algorithms are often seen as neutral, yet they can inherit the biases of their creators and the data they are trained on. This underscores the need for continuous evaluation and adjustment of the datasets and models used in predictive analytics.
To tackle bias, companies must implement techniques such as:
- Continuous monitoring of model outcomes across different demographic groups.
- Employing diverse teams in the data processing steps to incorporate various perspectives.
- Utilizing algorithms that are designed to be fair and reduce biases.
Institutions must recognize that predictive analytics holds the potential for both empowerment and discrimination; it’s a double-edged sword that demands careful wielding.
Transparency in Algorithm Usage
The second major ethical consideration revolves around transparency. How algorithms make decisions is often shrouded in mystery, leading to a situation referred to as the "black box" phenomenon. This lack of clarity can breed mistrust amongst users, clients, or anyone affected by these predictions. For individual investors, knowing how predictive models arrive at their conclusions is crucial for informed decision-making.
Transparency in algorithm usage can take several forms:
- Explainable AI: Creating models that are inherently interpretable by both technical and non-technical stakeholders.
- Documentation: Providing clear reports that outline how data was gathered, processed, and analyzed.
- Feedback Mechanisms: Establishing channels where users can ask questions about decisions made by algorithms, promoting dialogue and accountability.
Without transparency, stakeholders may feel powerless or skeptical about the data-driven decisions affecting their financial future. Thus, organizations that prioritize ethical considerations in predictive analytics will likely cultivate trust and long-lasting relationships with their clients.
In summary, navigating the ethical implications of predictive analytics is vital for its successful implementation. By addressing bias and discrimination issues and committing to transparency, businesses can not only enhance their models but also align their operations with ethical standards, ultimately fostering a more just and equitable data-driven future.
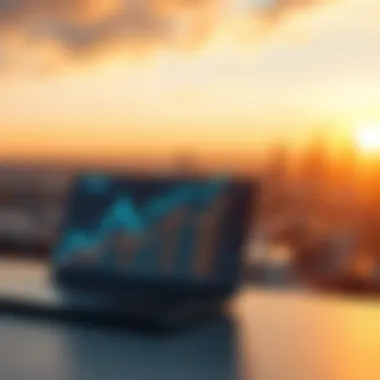
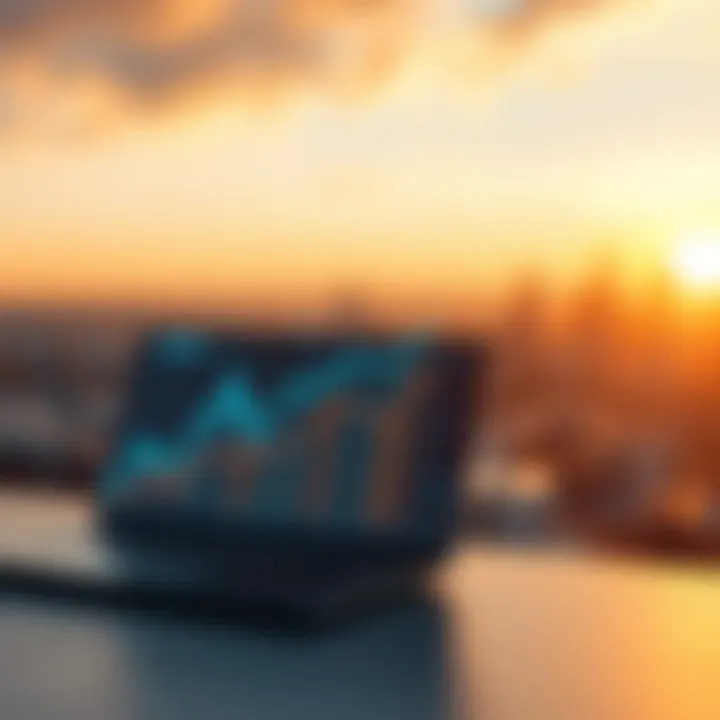
Future Trends in Predictive Analytics
Predictive analytics is constantly evolving and the future holds promising developments that can reshape how businesses and individual investors engage with data. Understanding these trends not only prepares organizations for the shifts ahead, but also enhances the utilization of predictive analytics for smart decision-making. The implications of these advancements are far-reaching, touching on multiple facets such as technology, data availability, and even societal impacts. In this section, we delve into two critical areas that are steering the course of predictive analytics into a new frontier.
Advancements in Machine Learning
Machine learning stands out as the backbone of predictive analytics today. Its continued evolution appears set to outpace the traditional analytical techniques, providing more precise and adaptable insights. For instance, algorithms that once needed human oversight can now operate autonomously by learning from real-time data inputs. Take, for example, how the financial sector employs reinforcement learning for algorithmic trading. The algorithms assess the market conditions instantaneously, learning from every trade they execute. This adaptability allows investors to seize fleeting opportunities where timing is everything.
Moreover, with the rise of deep learning, models can analyze vast amounts of unstructured data such as images, audio, and text to extract meaningful patterns that were previously inaccessible. Consider a scenario where predictive maintenance in manufacturing utilizes sensor data from machinery. The machine learning models can alert operators about potential failures before they occur, thus minimizing downtime and saving costs.
"In predictive analytics, the power of machine learning lies in its ability to continuously learn and adapt to new data, making it crucial for maintaining competitive advantage."
As machine learning techniques mature, the focus shifts toward the development of explainable AI, which emphasizes the transparency of algorithmic decisions. Investors will benefit from insights that demystify how predictions are made, fostering trust and making complex algorithms less of a black box.
Evolution of Data Sources
Our reliance on diverse data sources is growing. The traditional array of structured data from databases is being complemented by a rich tapestry of unstructured data—social media activity, online reviews, sensor data, and even IoT devices. This proliferation enhances predictive analytics, allowing a broader context for analysis. For individual investors, tapping into this diverse data pool could mean gaining an edge in understanding consumer behavior.
With advancements in edge computing, data processing can occur closer to the source, significantly reducing latency and enabling real-time analytics. Simply put, the speed of delivering insights is about to get turbocharged. In a fast-paced market where snap decisions count, harnessing data swiftly could be the difference between profit and loss.
As data availability expands, so do the ethical challenges surrounding it. Investors and businesses will need to navigate these waters carefully, ensuring that data privacy and consent remain paramount. The evolution of data sources might bring about more regulations to protect individual privacy, which in turn shapes how predictive analytics is implemented.
In summary, the future trends in predictive analytics point toward a landscape that is characterized by unprecedented advancements in machine learning and a rich evolution of data sources. Both elements hold the potential to refine how decisions are made and strategies are crafted—offering a world of opportunity for astute investors.
Adopting PAaaS for Individual Investors
Adopting Predictive Analytics as a Service (PAaaS) represents a significant shift for individual investors, especially those navigating the increasingly complex landscape of financial markets. With the rise of data-driven decision making, PAaaS offers tools that empower private investors to make informed choices. This section focuses on the essential elements, advantages, and considerations that surround the adoption of PAaaS, underlining its relevance in today’s investment climate.
Understanding Personal Investment Strategies
In the rapidly changing world of finance, personal investment strategies must adapt to both market fluctuations and emerging technologies. PAaaS enables individual investors to customize their investment approaches based on predictive insights generated from vast amounts of data. By leveraging machine learning algorithms and statistical models, investors can identify trends and patterns in the market that were previously elusive.
For instance, let’s say an individual investor is considering entering the tech sector. Using PAaaS, this investor can analyze historical data and forecast potential future performance based on various economic indicators. A comprehensive view like this can guide decisions about not just which stocks to purchase, but also when to enter the market for maximum benefit.
- Flexibility: Investors can tailor models to reflect their personal risk profiles, investment horizons, and portfolio goals.
- Real-time Data: With regular updates, investors have access to the most recent trends, potentially leading to decision-making that aligns with current market conditions.
- Scenario Analysis: PAaaS tools often support “what-if” analyses, allowing users to see the impact of different investment choices before committing capital.
Making Data-Driven Decisions
In an age where information is abundant, the challenge lies in discerning what data is relevant to making wise investment decisions. PAaaS facilitates this by providing a structured analytic approach that transforms raw data into actionable insights.
Investors can conduct deeper analyses by integrating data from multiple sources—ranging from market news to geopolitical developments. This comprehensive perspective aids in making decisions backed by data, rather than relying solely on intuition or gut feelings. Consider the following benefits:
- Enhanced Accuracy: By utilizing predictive models, investors can forecast outcomes with greater precision, minimizing the likelihood of expansive losses.
- Psychological Comfort: Data-driven decisions can alleviate the emotional burden often associated with investing, providing a sense of security.
- Portfolio Diversification Insights: PAaaS can highlight opportunities across various sectors that align with a typical investor's risk tolerance and investment strategy.
Investors who embrace data-driven decision-making may enjoy a distinct advantage—a robust portfolio that adapts to market changes and investor goals.
In summation, the adoption of PAaaS opens doors for individual investors to engage more intelligently with their portfolios. From understanding personal investment strategies to embracing data-driven decisions, PAaaS arms investors with the tools they need to navigate the ever-changing financial landscape.
Epilogue: Shaping the Future with PAaaS
Predictive Analytics as a Service (PAaaS) represents a significant shift in how organizations can approach decision-making. It's not merely a new tool; rather, it redefines the landscape of analytics, providing a more adaptable and scalable solution to meet contemporary demands. As this article has explored, the convergence of machine learning advancements and the democratization of data are paving the way for a future where insights are increasingly accessible. Organizations and individual investors stand to gain immensely from adopting PAaaS solutions that align with their strategic goals.
Recap of Key Insights
Reflecting on the various discussions within this article, a few key takeaways emerge that highlight the transformative potential of PAaaS:
- Flexibility and Scalability: Unlike traditional analytics, PAaaS can evolve with an organization's needs, making it easier to scale operations as data grows.
- Cost-Effectiveness: By outsourcing analytical capabilities through PAaaS, firms can significantly reduce the expense associated with maintaining in-house infrastructure.
- Enhanced Predictive Accuracy: With access to sophisticated algorithms and data, PAaaS enables better forecasting, which is vital for making informed business decisions.
- Fostering Innovation: PAaaS encourages the use of cutting-edge technologies, allowing businesses to stay ahead of competitors by leveraging new analytic techniques.
Ultimately, these insights paint a picture of a future where predictive analytics becomes standard, allowing organizations to unlock value and drive growth through data-driven strategies.
Encouraging Smart Adoption
For those considering diving into the world of PAaaS, it's crucial to approach adoption thoughtfully. Here are some strategies to make the transition smoother:
- Assess Your Needs: Before selecting a PAaaS provider, take a hard look at what your organization specifically needs. Are you focusing on customer behavior, operational efficiency, or perhaps risk management? Knowing this will help guide your choice.
- Choose Wisely: Not all PAaaS offerings are created equal. Look for providers with a proven track record in your industry and seek out reviews or case studies that showcase their success.
- Start Small: If possible, piloting PAaaS solutions on a smaller subset of data can help refine processes before full-scale implementation. This approach can mitigate risk and provide valuable learnings.
- Train and Prepare Your Teams: Ensuring that the teams involved in utilizing PAaaS are adequately trained is pivotal. This step not only optimizes the use of the service but also fosters a culture of analytical thinking within your organization.
Leveraging PAaaS can indeed reshape the future landscape of analytics, driving impactful decision-making. As the analytics domain continues to evolve, those who adapt smartly will likely find themselves ahead of the curve.